Scriptieprijs voor onderzoek over oplaadstations e-auto’s
Von Rüden rondde zijn sciptie af aan de Rotterdam School of Management van de Erasmus Universiteit Rotterdam. Hieronder een interview met hem over zijn bevindingen.
Could you tell us briefly what your thesis is about?
My thesis is about how to make right decisions about public infrastructure, particularly about placing charging stations for electric sharing vehicles. I wanted to predict where charging station providers should place their stations so that they can run a profitable business and offer sufficient charging stations, which in turn can lead to an increase in the use of electric sharing vehicles. I made my predictions based on data from two electric car sharing companies, Car2Go and DriveNow, in the cities of Amsterdam, Berlin, Copenhagen, Hamburg and Munich.
How are decisions about where to place charging stations currently made?
Decisions about where to place charging stations are often made by public parties and private enterprises together, but based on gut feelings. For instance, they would look at where there are a lot of cars passing by or at supermarket parking lots where people often spent at least half an hour and thus have time to charge their cars. Then they would place their charging stations at some of those locations. But doing it this manual way is not necessarily a good way. Some places may be overlooked and it’s also a very time-consuming approach.
Could you tell us a bit more about how your approach might be more effective than the manual way, and which problems it could solve?
There is a chicken-egg problem in discussions about electric vehicle charging stations. On the one hand, if someone would consider buying an electric vehicle, he or she will probably be afraid of running out of battery. That’s what’s called range anxiety. Due to such anxiety people would be more inclined to buy a car if there are a lot of charging stations. On the other hand, if there are not enough electric cars on the road, charging station providers would think it’s not profitable to place a lot of charging stations if there aren’t a lot of cars on the road. So, to some extent, car users wait for the station providers to place stations, and station providers wait for car users to buy and use cars. The instrument that I built can help overcome this problem, by predicting what the best places are to place charging stations.
At the same time, the instrument I developed also has larger societal and environmental significance. Large cities have too much cars. There are a lot of traffic jams, which lead to a lot of pollution. My instrument could hopefully reduce those problems by optimizing car sharing station placing and thus car sharing usage.
Is it possible to estimate the effect of electric car sharing on solving those problems more specifically?
That’s something I haven’t looked at in my research, because that wasn’t the focus. But if we have data on traffic and electric vehicle sharing in a particular city as well as pollution and traffic jam levels then that effect would certainly be measurable empirically. In fact, there have been some other research projects across the globe that already show that car sharing can reduce pollution and traffic.
So it seems that your instrument could contribute to solving a significant problem. Haven’t there been other people before you that tried to develop a similar instrument for pinpointing the best charging station locations?
To my knowledge, my research hasn’t been done before in the same way and as extensively as I did. There was another student before me that had a somewhat similar interest and took a somewhat similar approach, but she focused only on the city of San Diego. There has also been some research done about how people are using existing car sharing services and how that usage could be optimized, but that research did not look into how charging stations could be placed more optimally.
Is your instrument now fully developed and ready to use by governments and companies, or would you recommend further research before actually using it in policy-making?
What I have developed can already be used for first decisions, specifically to support decisions, rather than to make decisions. Generally, my model seems to make quite smart suggestions, but it might also suggest completely ridiculous locations. So it should be used as support for decision-making.
The interesting thing is that if companies and governments use it, they would probably have access to much more data, such as data about actual revenues. With such data, it would be possible to further refine my model.
So if your instrument is already functional, is there then still a reason why commercial enterprises or governments would not want to work with it?
There are two possible barriers. First, technology-wise, it’s not that hard to develop an instrument like I did. But still companies and governments need the skills to develop and work with such an instrument, and that’s not necessarily the case. Everybody talks about Big Data and machine learning, but only when you work with it you can truly understand their power and what they mean, but also their limitations.
Second, and related, priorities may not yet be focused sufficiently on using instruments like mine. Decision-makers might not have looked into them sufficiently, for instance because they see them as black boxes.
The good news is that the data which I worked with is publicly accessible and are not about individual persons. In other words, I don’t know who drove in which car from where to where and when. So my instrument does not raise concerns about privacy or security of data, which otherwise would form an obstacle for governments or companies to actually use it. Of course it would be possible to use more personalized data in the instrument, which might optimize the prediction of best locations for charging stations, but then the instrument would also become more sensitive and difficult to use.
Did you try to stimulate the public or commercial use of your instrument yourself?
No, I haven’t. When I finished my study I travelled for a while and then I started my new job as a data scientist at SAP, so I didn’t have any time to further work with it. However, I do still think it’s a very interesting topic, and I still think it’s something worth pursuing. My hope is that other people will pick up the idea and try to implement it. For instance, some of the charging station operators I talked with were very interested in my research and said they will consider using my instrument in their decision-making.
U kunt de scriptie hier downloaden.
Over Urban Big Data Rotterdam
Auteur: Fadi Hirzalla, Erasmus Universiteit Rotterdam
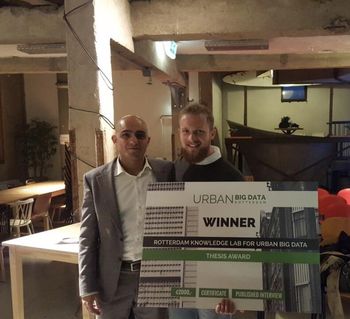
Jonathan von Rüden neemt zijn prijs van 2000 euro in ontvangst
Reactie plaatsen •